Bioinformatics
Bioinformatics is an interdisciplinary field that develops methods for understanding biological data, in particular when the data sets are large and complex.
According to Dr. Farrugia, Gianrico, M.D. - President and CEO, Mayo Clinic, Rochester , Minnesota, ; "In the absence of basic/discovery science we will not have medical breakthroughs. Indeed at Mayo Clinic, we not only have basic science departments but also have many of PhDs in our clinical departments. It is indeed why Mayo Clinic has the number one ranking for clinical care – we integrate research, education and clinical care."
Biomimicry Cognitive Network Design Approaches
Overview: In collaboration with neurologists, the goal is to perform physical modeling of neural networks to better understand Cognitive Network Design Approaches, based on how Human Brain functions.
This interdisciplinary research has the potential to revolutionize how we design communication networks by incorporating principles from biological systems. By understanding and emulating the brain's network dynamics, we could develop more resilient and efficient communication systems. Furthermore, the project's insights into brain capacity modeling could have broader applications in neuroscience and cognitive science.
In (White Cloud Express, 13) the author Jay Harman [1] interviewed Dr. Kavehrad on a paper he had published that was funded through a DARPA/AFRL project [2]. Dr. Kavehrad had observed that dolphin chirps look very similar to a wavelet waveform and he used this observation to transmit ultrashort optical pulses through clouds with promising results. Dr. Kavehrad now believse that the next revolution in networking will take a similar course. Therefore, this research is to verify the theoretical concepts introduced in the methodology section below, using neural network simulators. This potentially creates a new frontier for the research on human brain. Stable, persistent activity has been thought to be important for neural computations and it may underlie short-term memory.
Researchers at Cambridge Univ. 1989, following work on attractors in artificial neural networks, suggested that persistent neural activity in biological networks is a result of dynamic attractors in the state space of recurrent biological networks. This seminal work resulted in attractor networks becoming a mainstay of theoretical neuro-science. More recent work has relied largely on spiking models, though of varying degrees of biological plausibility. In addition, there is increasing evidence that many brain areas act as attractor networks. Here, we introduce system-level, physically detailed modeling tools for photonic neural networks, and use them to study the behavior of attractor networks.
Cognitive Network
Methodologies: Human Brain Capacity "C" is defined by:
In the proposed research, Dr. Kavehrad verifies this theory through a series of experiments [3]. The formula above demonstrates the concept and for a recent summary presentation, see [4].
In this equation; Intelligence Quotient (IQ) is what a human being is born with and is a matter of heredity. Human brain’s capacity grows linearly as a function of IQ.
Parameter “A” is the ratio of (Rational Mind and Emotion) to (Irrational Mind and Emotion) which is related to the human Emotional Intelligence (EQ). We call this"Wisdom". Wisdom is Epigenetic and modifiable in time; meaning it can, for example, grow with experiences, travel and association with wise and experienced people. However, the growth of Brain Capacity as a function of Wisdom is slow and logarithmic in this model. The mind capacity can grow with “A” logarithmically. Thus, when "A" is very small, "C" is nearly zero (babies at birth).
In general, an attractor network is a network of nodes (i.e., neurons in a biological network), often recurrently connected, whose time dynamics settle to a stable pattern. The particular pattern a network settles to is called its ‘attractor’. In theoretical neuro-science, different kinds of attractor neural networks have been associated with different functions, such as memory, motor behavior, and classification.
- Jay Harman, "The Shark's Paintbrush: Biomimicry and How Nature is Inspiring Innovation," White Cloud Press, ISBN: 978-1-935952-84-8, 2013.
- M. Kavehrad, "Mitigation Approaches for Optical Imaging Through Clouds and Fog,” DARPA/AFRL Final Report: AFRL-RY-WP- TR-2009-1331, Released on December 9, 2009.
- VIDEO: Neuroplasticity of Human Brain
- M. Kavehrad, "Biomimicry-Cognitive-Network-Design," Recent Summary, April 2021.
Medical Flow Cytometry
The objective is to improve the capabilities of the state-of-the-art flow cytometers in terms of recognition of cell characteristics.
Flow cytometry is used to count and analyze the size, shape and properties of individual cells within a heterogeneous population of cells. Flow cytometry data is quantitative and can be analyzed in depth by specific flow cytometry software programs. Once a suspension of individual cells has been prepared for flow cytometry analysis from cells, tissues, or organisms, the prepared sample is placed on the flow cytometer. How does a flow cytometer analyze the size, shape, and properties of individual cells? A flow cytometer contains several key components, including the sample, fluidics that move the sample into the flow cytometer, lasers, optics which gather the light, detectors to sense the light, and a computer system to output the data into a form that can be analyzed by the researcher. In general, this is a Biochemical Sensing approach, as shown in Fig.-1 below.
The global flow cytometry market size is projected to grow from an estimated USD 4.0 billion in 2019 to USD 6.4 billion by 2025, at a CAGR of 8.3% during the forecast period. Market growth is largely driven by factors such as technological advancements in flow cytometers, the increasing adoption of flow cytometry techniques in research activities and clinical trials, growing focus on immunology and immuno-oncology research, increasing incorporation of AI platforms in flow cytometry workflows and advancements in flow cytometry software, high incidence and prevalence of HIV-AIDS and cancer, and the increasing availability of novel application-specific flow cytometry products. The growing market penetration in emerging countries, growth in stem cell research, adoption of recombinant DNA technology for antibody production, and evolution of tandem flow cytometry technologies are expected to present a wide range of growth opportunities for market players.
-------------------------------------------------
Medical Imaging Feasibility in Body Fluids using Markov Chains
Overview: In collaboration with arthroscopy surgeon Dr. A.D. Armstrong (MD) Medical Imaging as pertains to aurthoroscopy is being investigated.
A relatively wide field-of-view and high resolution imaging is necessary for navigating the scope within the body, inspecting tissue, diagnosing disease, and guiding surgical interventions. As the large number of modes available in the multimode fibers (MMF) provides higher resolution, MMFs could replace the millimeters-thick bundles of fibers and lenses currently used in endoscopes. Endoscopy is a nonsurgical procedure that allows examining person’s inside body by inserting a device directly to target part of the body. Endoscope is a medical imaging device that looks like a tube and has a camera, light source and controllers at one end and has objective lens and internal instrument set at another end. General structure is shown in Fig. - 1.
Figure-1a General Structure of Endoscope. Figure-1b General Structure of Medical Imaging.
Figure-1c Medical Imaging and Optical Lenses.
Nowadays, endoscope is used for different procedures, and some examples are laparoscopy, rhinos-copy, and arthroscopy. Endoscopy allows obtaining medical imaging directly from the organ with only small incision which makes procedure less invasive and patients can recover easily. MMF is considered as a strongly aberration environment. Light that is propagated through MMF is a subject to be distorted. At the output of MMF, because of higher pulse spreading rates, phases of modes tend to expand and thus due to interference they become distorted. By using spatial light modulation (SLM) it is possible to shape wave-front of the light at the distal end, and receive at the proximal end, output of MMF to detector, corrected light propagation SLM has been used for SMF spatial wave-front modulation where manipulation of spatial and spectral properties of the waves was achieved. In case of MMF, where multiple numbers of modes should be modulated, the approach was achieved by using computational algorithm in the reciprocal space. Spatial phase modulation is achieved by increasing the intensity. In other words, at each point (pixel) only certain
modes contribute energy to the average intensity, and by doing so iteratively the intensity increases and makes the spot brighter. Then, by combining all the holograms of each point on the object, we can obtain the entire picture of the object.
However, attributes of body fluids and obscurants such as blood, impose perennial limitations on resolution and reliability of optical imaging inside human body. To design and evaluate optimum imaging techniques that operate under realistic body fluids conditions, a good understanding of the channel (medium) behavior is necessary.
In most prior works, Monte-Carlo Ray Tracing (MCRT) algorithm has been used to analyze the channel behavior. This task is quite numerically intensive. The focus of this paper is on investigating the possibility of simplifying this task by a direct extraction of state transition matrices associated with standard Markov modeling from the MCRT computer simulations programs. We show that by tracing a photon’s trajectory in the body fluids via a Markov chain model, the angular distribution can be calculated by simple matrix multiplications. We also demonstrate that the new approach produces result that are close to those obtained by MCRT and other known methods. Furthermore, considering the fact that angular, spatial, and temporal distributions of energy are inter-related, mixing time of Monte-Carlo Markov Chain (MCMC) for different types of liquid concentrations is calculated based on Eigen-analysis of the
state transition matrix and possibility of imaging in scattering media are investigated. To this end, we have started to characterize the body fluids that reduce the resolution of imaging .
- Akhanova A, Kavehrad M., Deng P., and Armstrong A.D., “Analysis of Medical Imaging Feasibility in
Presence of Body Fluids Using Markov Chains,” Proceedings of Drug Discovery and Therapy World congress,
Boston-Mass, August 2016. - M. Kavehrad and A.D. Armstrong, “Medical Imaging Feasibility in Body Fluids Using a Markov Chains,” Proceedings of SPIE Photonics West, San Francisco-CA., January 2017.
- Z. Hajjarian, M. Kavehrad and J. Fadlullah, ”Analysis of Wireless Optical Communications Feasibility in Presence of Clouds Using Markov Chains,” IEEE JOURNAL ON SELECTED AREAS IN COMMUNICATIONS, VOL. 27, NO. 9, DECEMBER 2009.

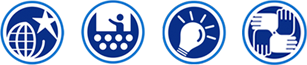